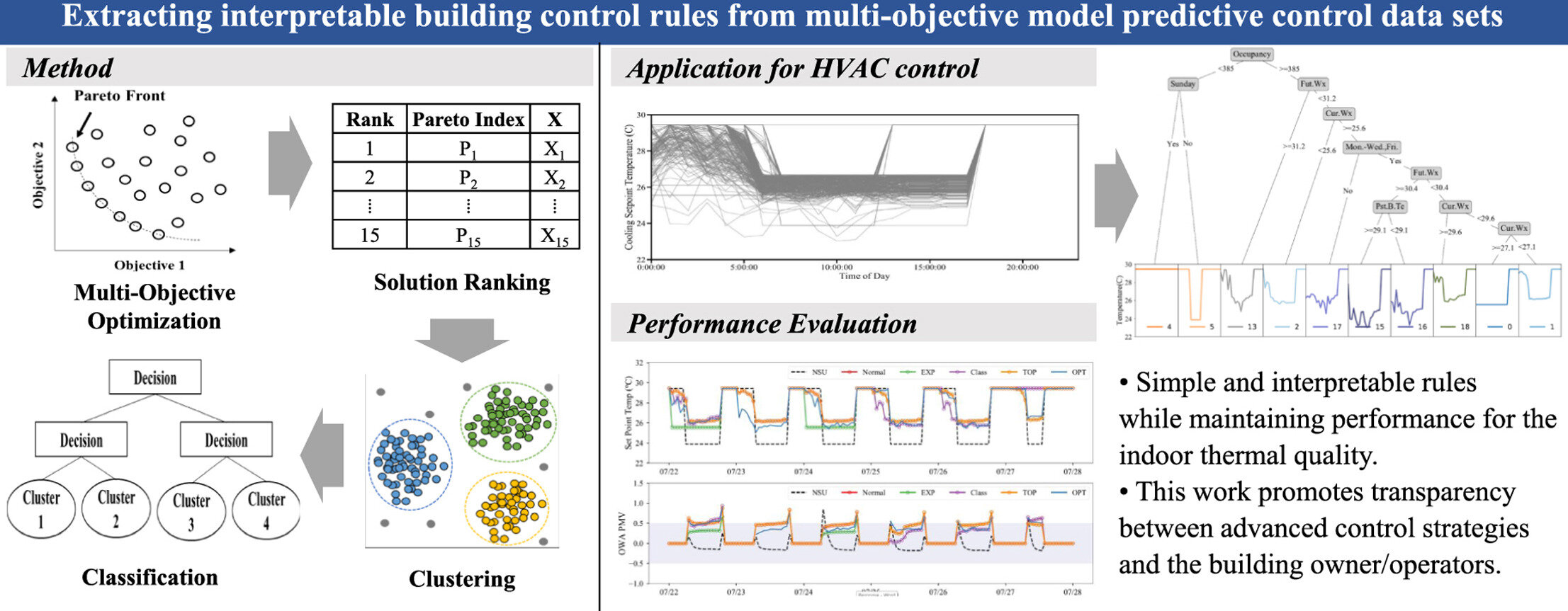
Energy (2021). DOI: 10.1016/j.energy.2021.122691″ width=”800″ height=”312″/>
Graphical abstract. Credit: Energy (2021). DOI: 10.1016/j.energy.2021.122691
Control mechanisms for heating, ventilation and air conditioning in buildings follow set parameters to make conditions in a building more comfortable, but what they save on time can reduce efficiency and increase energy costs, according to Gregory Pavlak, assistant professor of architectural engineering. More sophisticated control models, known as model predictive controllers, can optimize multiple variables to save on energy, operating costs and carbon emissions but can require much more time to find solutions.
Penn State researchers developed a method that leverages machine learning to create controls that balance building energy cost, comfort and efficiency while computing at a fast pace. They published their findings in Energy in February.
“Detailed model predictive controllers may not be able to compute solutions fast enough for real-time operations in some buildings,” Pavlak said. “We used machine learning to generate a simple, easily interpretable set of rules for reducing building cooling energy and operating costs—without needing to run model predictive controllers in real time.”
To identify shortcuts to the optimal solutions made possible by model predictive controllers, the researchers used the controllers and collected data from them. The team targeted patterns that consistently led to good performance. Once these patterns were identified, the researchers filtered and grouped them into categories of control strategies. These categories provided examples of high-performance control strategies that could be used to train a machine learning model. Classification trees, which generate a set of decision rules, were used for the machine learning model to determine the best times of day to cool a building.
These rules were simple enough to work with basic control hardware, as well as easy to interpret, according to Pavlak.
“Once these rules have been generated, they work extremely fast and can be implemented in low-cost controllers and standard building automation equipment,” he said. “They’re also very interpretable. Operators and engineers can read the rules and easily understand how the systems will behave.”
The rules based on the data from model predictive controllers attained energy efficiency and energy cost levels comparable to those of a model predictive controller in action. The best rule sets attained 95–97% of the energy savings and 89–92% of the cost objective savings of the detailed model predictive controller. These values were reached through significantly faster computations: To schedule a control strategy for one day, the original model predictive controller required several hours of computation, while the researchers’ method could complete the task in less than a second.
The researchers aim to enable more sophisticated explorations of this technique with future research, including investigations on a variety of buildings, HVAC systems and operating conditions.
Deep learning cuts costs in building control
More information:
Min Gyung Yu et al, Extracting interpretable building control rules from multi-objective model predictive control data sets, Energy (2021). DOI: 10.1016/j.energy.2021.122691
Provided by
Pennsylvania State University
Citation:
Machine learning offers shortcut to optimal HVAC operation (2022, March 4)
retrieved 4 March 2022
from https://techxplore.com/news/2022-03-machine-shortcut-optimal-hvac.html
This document is subject to copyright. Apart from any fair dealing for the purpose of private study or research, no
part may be reproduced without the written permission. The content is provided for information purposes only.